Revolutionizing Healthcare: The Synergy of Knowledge Graphs and Large Language Models
The following is a guest article by Ben Cushing, Chief Architect, Health & Life Sciences at Red Hat In the evolving landscape of healthcare, the fusion of knowledge graphs (KGs) and large language models (LLMs) is redefining how clinicians, patients, and data systems interact. This emerging alliance is transforming not only the way healthcare professionals […]
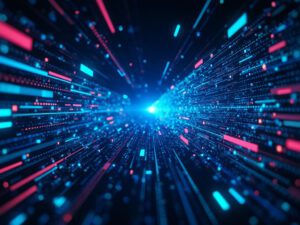
The following is a guest article by Ben Cushing, Chief Architect, Health & Life Sciences at Red Hat
In the evolving landscape of healthcare, the fusion of knowledge graphs (KGs) and large language models (LLMs) is redefining how clinicians, patients, and data systems interact. This emerging alliance is transforming not only the way healthcare professionals access and interpret information, but also how patients receive care in an increasingly data-driven world. Let’s examine how, as healthcare organizations grapple with the complexities of big data, the combination of knowledge graphs and large language models offers a new frontier in efficiency, personalization, and clinical decision-making.
The Power of Combining Knowledge Graphs and LLMs
In today’s data-saturated world, healthcare stands at a crossroads. While digitization has brought about an unprecedented influx of information – electronic health records, medical research, insurance data, and more – the ability to effectively use that data in clinical settings has lagged. Now, a breakthrough is emerging from the intersection of artificial intelligence and healthcare: the union of knowledge graphs and large language models.
A knowledge graph is a structured representation of information, often visualized as nodes (entities such as diseases, treatments, or patient observations) connected by edges (relationships). More than a visualization tool, KGs are sophisticated data models that support powerful querying, enabling clinicians to extract contextually rich insights from vast biomedical databases. Meanwhile, large language models are trained on immense amounts of human language data to understand, interpret, and generate human-like text. In healthcare, LLMs can interpret clinical language, summarize patient records, draft medical reports, and assist in answering complex questions with speed and precision.
These technologies are impressive enough on their own. But when combined, the synergy of the two unlocks transformative capabilities for how clinicians make decisions and patients experience care. Imagine a doctor asking a clinical question – perhaps about the best treatment for a patient with both diabetes and heart failure – and instantly receiving an answer that’s been synthesized from the patient’s full medical record, current clinical guidelines, and the latest research. Such capabilities aren’t just theoretical; they’re becoming a reality through the combination of KGs and LLMs.
Transformative Capabilities
By integrating KGs with LLMs, the healthcare system gains the best of both worlds: structured, explainable relationships that feed into language models capable of interpreting and articulating those relationships in real time. The combined technologies are structuring massive amounts of biomedical data – everything from research publications to patient histories – into an interconnected framework for a system that doesn’t just retrieve data, but also understands and renders it in a meaningful, context-rich way.
Most current AI applications in healthcare rely on vector databases – collections of coordinates representing data in a way that facilitates similarity search, such as matching terms like “sutures” and “stitches”. Here’s where KG-LLM systems excel. Unlike vectors, the KG maintains explicit contextual and relation reasoning that the LLM can then use to interpret and generate highly accurate, context-aware responses – in this example, referencing “stitches” in patient-focused communications and “sutures” in physician-focused guidelines.
The result is a powerful hybrid framework that not only understands clinical language but also “knows” the logic behind the medical data. This framework serves as the foundation to translate data into readable, actionable insight. It’s a deeply collaborative process, with engineers, data scientists, and clinicians working hand-in-hand to ensure the graphs reflect real-world medical logic and the LLMs generate reliable and highly contextualized outputs.
Powerful Real World Applications
Nowhere is the combination of KGs and LLMS more valuable than in the fast-paced environment of clinical care. Physicians often face information overload, juggling time constraints with the need to make complex decisions. Traditionally, they might have to manually dig through EHRs or clinical guidelines. But with KGs and LLMs working together, they can simply pose a question, as they would to a colleague, and receive an immediate, personalized, and evidence-based response.
Another promising application is in the world of prior authorization. Insurance providers frequently deal with automated systems that lack the contextual understanding needed to approve payment. This can lead to denials by default. But a system powered by KGs and LLMs can pull in all the necessary data, evaluate it with context, and deliver a justified recommendation “yes” or “no” in real time, potentially reducing delays in treatment and frustration for both providers and patients.
Additional areas for transformation are clinical trial matching. Traditionally, identifying eligible patients for research studies has been a time-consuming process requiring manual reviews of patient data against trial criteria. A KG-LLM system can automatically scan records and match patients to appropriate trials based on detailed eligibility factors, accelerating recruitment and expanding access to cutting-edge therapies.
Furthermore, the combination of KGs and LLMs in pharmacology is helping researchers repurpose existing drugs for new conditions or predict adverse drug reactions before they happen. And in the realm of chronic disease management, KG-LLM systems can be used to design smarter nutrition plans, identify comorbidities earlier, and guide long-term care with a more holistic understanding of patient data.
Conclusion
The healthcare industry has long struggled with the “data paradox” of plentiful information and limited ability to manage it effectively. KGs and LLMs represent a critical solution in allowing healthcare teams to not just store and retrieve data, but also understand and act upon it. For healthcare professionals, this means greater confidence and clarity in decision-making. For patients, it means care that’s more personalized, timely, and aligned with the full picture of their health. And for the entire system, it’s a pathway to greater efficiency and smarter resource use.