Mount Sinai Calibrates AI Algorithm to Provide Specific HCM Risk Probabilities from ECGs
What You Should Know: – Researchers at Mount Sinai have enhanced an existing artificial intelligence (AI) algorithm to provide specific numeric probabilities identifying patients at risk for hypertrophic cardiomyopathy (HCM), a common inherited heart condition, based on their electrocardiogram (ECG) readings. – The calibration of the FDA-approved Viz HCM algorithm, detailed in a study published ... Read More
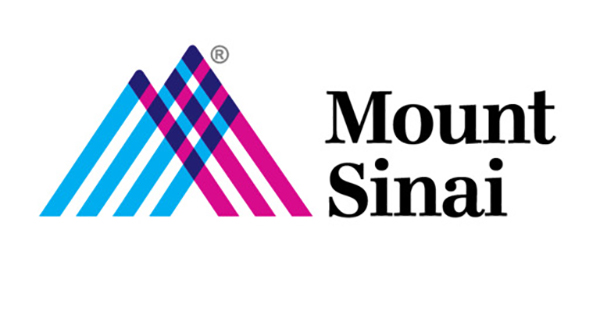
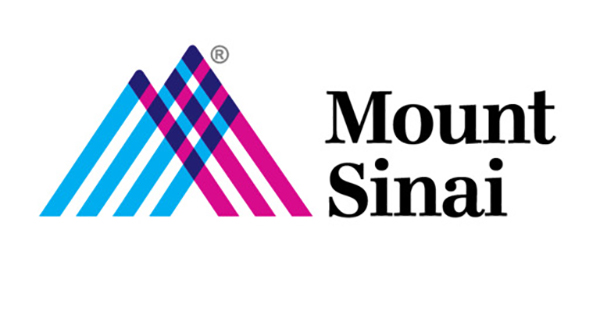
What You Should Know:
– Researchers at Mount Sinai have enhanced an existing artificial intelligence (AI) algorithm to provide specific numeric probabilities identifying patients at risk for hypertrophic cardiomyopathy (HCM), a common inherited heart condition, based on their electrocardiogram (ECG) readings.
– The calibration of the FDA-approved Viz HCM algorithm, detailed in a study published April 22, 2025, in NEJM AI, aims to provide more meaningful and actionable information to both clinicians and patients.
Moving Beyond Flags to Personalized Risk Probabilities
While the original Viz HCM algorithm could identify an ECG as potentially indicative of HCM, the Mount Sinai calibration adds a crucial layer of specificity. Instead of receiving a general alert such as “flagged as suspected HCM” or “high risk of HCM,” clinicians and patients can now understand the risk quantitatively. “For example… the Mount Sinai study allows for interpretations such as, ‘You have about a 60 percent chance of having HCM’,” explained corresponding author Dr. Joshua Lampert, Director of Machine Learning at Mount Sinai Fuster Heart Hospital and Assistant Professor of Medicine (Cardiology, and Data-Driven and Digital Medicine) at the Icahn School of Medicine at Mount Sinai. This refinement offers patients who may not have been previously diagnosed a clearer, more individualized understanding of their potential risk.
Study Validates Calibrated AI Model Accuracy
To develop and validate this calibration, the Mount Sinai research team applied the Viz HCM algorithm to ECG data from nearly 71,000 patients obtained between March 7, 2023, and January 18, 2024. The algorithm flagged 1,522 of these ECGs with a positive alert for potential HCM. The researchers then conducted detailed reviews of patient medical records and imaging data to confirm which of these flagged individuals had a verified HCM diagnosis. Using this confirmed outcome data, they applied model calibration techniques to the AI algorithm’s output scores. The study found that the resulting calibrated model successfully provided an accurate estimate of a patient’s actual likelihood of having HCM.
Addressing an Underdiagnosed Cardiac Condition
Hypertrophic cardiomyopathy affects an estimated one in 200 people worldwide and is a leading cause of heart transplantation. However, because many patients remain asymptomatic until the disease is advanced, it often goes undiagnosed. Tools like the calibrated Viz HCM algorithm offer promise for earlier detection and intervention. The Mount Sinai team plans to expand this research and test the AI calibration approach for HCM in additional health systems across the country to determine if the strategy is universally applicable.
Improving Patient Triage, Counseling, and Engagement
The ability to assign specific probabilities has significant potential clinical benefits. “This is an important step forward in translating novel deep-learning algorithms into clinical practice by providing clinicians and patients with more meaningful information,” stated Dr. Lampert. He elaborated that “Clinicians can improve their clinical workflows by ensuring the highest-risk patients are identified at the top of their clinical work list using a sorting tool.” Furthermore, more precise risk information enhances patient counseling. “Patients can be better counseled by receiving more individualized information through model calibration,” Dr. Lampert added. This improved communication can lead to faster, more personalized evaluations and treatment initiation, potentially preventing severe HCM complications like sudden cardiac death, especially in younger individuals. “This can transform clinical practice because the approach provides meaningful information in a clinically pragmatic fashion to facilitate patient care,” he concluded.
Expert Insights on Pragmatic AI Implementation in Cardiology
Senior authors on the study emphasized the importance of translating AI advancements into practical clinical tools. “This study provides much-needed granularity to help rethink how we triage, risk-stratify, and counsel patients,” said Dr. Vivek Reddy, Director of Cardiac Arrhythmia Services for the Mount Sinai Health System. “Using hypertrophic cardiomyopathy as an illustrative use case, we show how we can pragmatically operationalize novel tools even in the setting of less common diseases by sorting AI classifications to triage patients.” Dr. Girish N. Nadkarni, Chair of the Windreich Department of Artificial Intelligence and Human Health at Icahn Mount Sinai, added, “This study reflects pragmatic implementation science at its best… It’s not just about building a high-performing algorithm—it’s about making sure it supports clinical decision-making in a way that improves patient outcomes and aligns with how care is actually delivered. This work shows how a calibrated model can help clinicians prioritize the right patients at the right time.”