Breaking Data Barriers: Can Anthropic’s Model Context Protocol Enhance AI Performance?
Anthropic’s innovative Model Context Protocol (MCP) aims to tackle fragmented data and boost the efficiency of AI-powered solutions. Could it become the standard for context-aware AI integration? One of the most pressing challenges in artificial intelligence (AI) innovation today is large language models’ (LLMs) isolation from real-time data. To tackle the issue, San Francisco-based AI […] The post Breaking Data Barriers: Can Anthropic’s Model Context Protocol Enhance AI Performance? appeared first on Unite.AI.
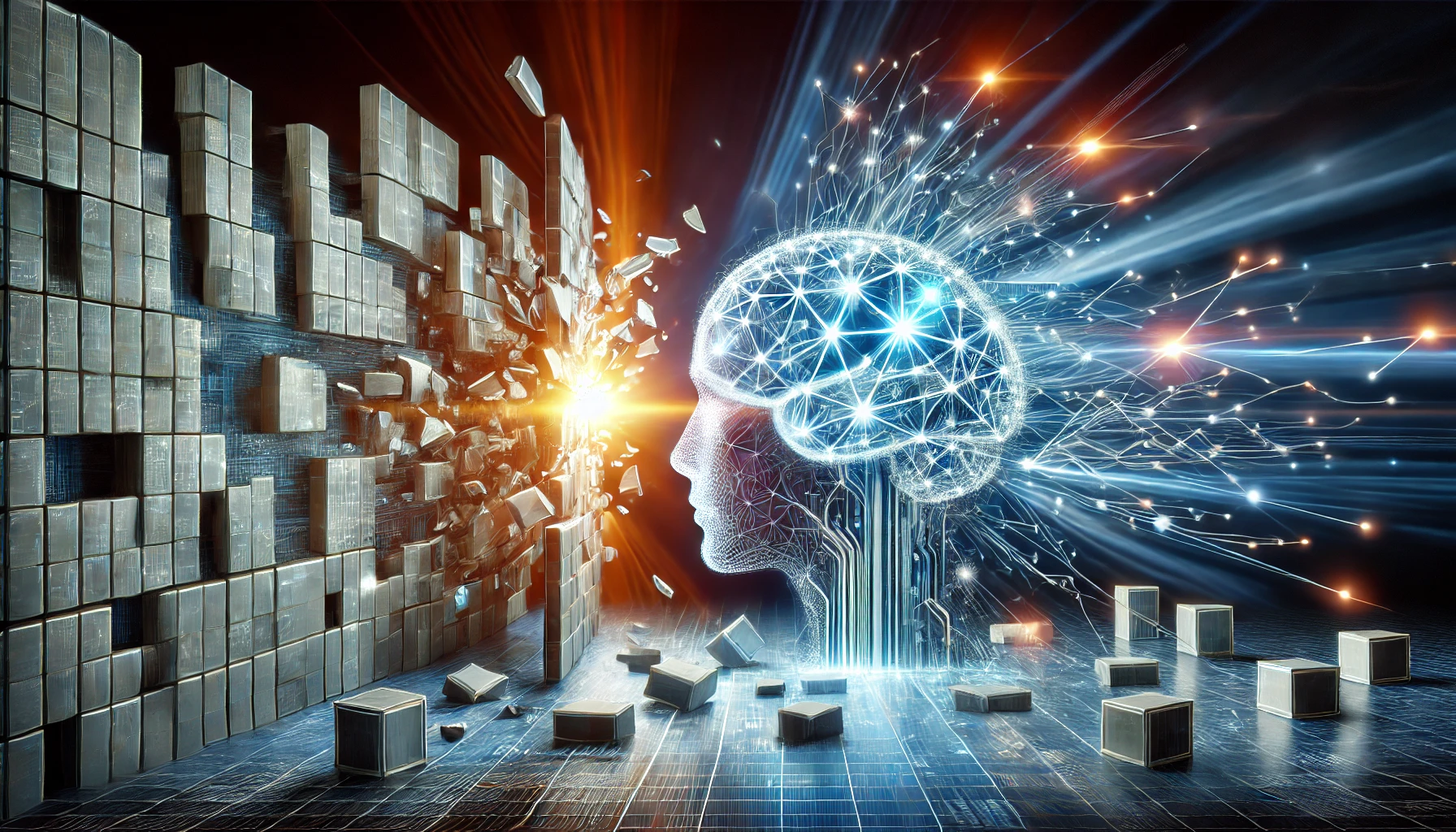
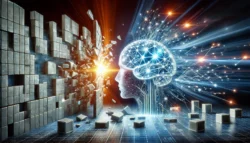
Anthropic’s innovative Model Context Protocol (MCP) aims to tackle fragmented data and boost the efficiency of AI-powered solutions. Could it become the standard for context-aware AI integration?
One of the most pressing challenges in artificial intelligence (AI) innovation today is large language models’ (LLMs) isolation from real-time data. To tackle the issue, San Francisco-based AI research and safety company Anthropic, recently announced a unique development architecture to reshape how AI models interact with data.
The company’s new Model Context Protocol (MCP), launched as an open-source project, is designed to boost the efficiency of AI through a “two-way communication between AI-powered applications and realtime, diverse data sources.”
The architecture is built to address a growing frustration: outdated AI outputs caused by a lack of connection to real-time data. Anthropic claims that the unified protocol can enhance AI development and functionality for businesses, and make them more human-like through real-time context awareness. According to the company, every new business data source requires custom AI implementations, creating inefficiencies. MCP seeks to address this by offering a standardized framework that developers can adopt universally.
“The architecture is straightforward: developers can either expose their data through MCP servers or build AI applications (MCP clients) that connect to these servers. Instead of maintaining separate connectors for each data source, developers can now build against a standard protocol,” Anthropic explained in a blog post. “As the ecosystem matures, AI systems will maintain context as they move between different tools and datasets, replacing today's fragmented integrations with a more sustainable architecture.”
AI models, including but not limited to Anthropic’s flagship assistant Claude, can integrate with tools like Google Drive, Slack, and GitHub. Experts suggest that MCP has the potential to transform business AI integrations in the same way Service-Oriented Architecture (SOA) and other protocols revolutionized application interoperability.
“Having an industry-standard protocol for data pipelines between LLMs and data sources is a game changer. Similar to REST and SQL in the software industry, standardized protocols such as MCP can help teams build GenAI applications faster and with better reliability,” Gideon Mendels, co-founder and CEO of AI model evaluation platform Comet, told me. “This follows the market realization in the last six months that a great LLM model is not enough.”
Anthropic also revealed that early enterprise adopters including Block and Apollo have already integrated MCP into their systems. Meanwhile, development tool providers such as Zed, Replit, Codeium, and Sourcegraph are collaborating with MCP to enhance their platforms. This partnership aims to help AI models and agents retrieve more relevant information through real-time data, grasp context more effectively, and generate nuanced outputs for enterprise tasks such as coding, with greater efficiency.
“AI models that are more human-like and self-aware can make the technology feel relatable, which could drive wider adoption,” Masha Levin, Entrepreneur in Residence at One Way Ventures, told me. “There's still a lot of fear around AI, with many seeing it as merely a machine. Humanizing these models could help ease those fears and foster smoother integration into everyday life.”
Levin also cautioned about a potential downside. “There’s a risk that businesses may become overly reliant on AI for support, allowing it to influence their decisions in extreme ways, which could lead to harmful consequences.”
However, the true test for MCP will be its ability to gain widespread adoption and outpace its competitors in a crowded market.
Anthropic MCP vs. OpenAI and Perplexity: The Battle for AI Innovation Standards
While Anthropic MCP’s open-source approach marks a notable advancement for AI innovation, it enters a competitive landscape dominated by tech giants like OpenAI and Perplexity.
OpenAI’s recent “Work with Apps” feature for ChatGPT showcases similar capabilities, although with a proprietary focus on prioritizing close partnerships over open standards. This feature allows ChatGPT to access and analyze data and content from other apps—but only with user permission, eliminating the need for developers to manually copy and paste. Instead, ChatGPT can review the data directly from an app, delivering smarter, context-aware suggestions due to its integration with real-time internet data.
Moreover, the company also introduced its real-time data architecture in October, called the “Realtime API,” which enables voice assistants to respond more effectively by pulling in fresh context from the internet. For instance, a voice assistant could place an order on a user’s behalf or retrieve relevant customer information to deliver personalized responses. “Now with the Realtime API and soon with audio in the Chat Completions API, developers no longer have to stitch together multiple models to power these experiences,” OpenAI said in a blog post. “Under the hood, the Realtime API lets you create a persistent WebSocket connection to exchange messages with GPT-4o.”
Likewise, Perplexity’s real-time data protocol for AI, known as the “pplx-api,” provides developers with access to its large language model (LLM). This API allows applications to send natural language queries and receive detailed, real-time information from the web. Through a single API endpoint, it enables up-to-date data retrieval and context-aware responses for AI applications, empowering developers to build applications that remain aligned with the latest information.
“Typically, the industry tends to standardize on one open source solution, but often that takes years. It’s very likely that OpenAI will try to introduce more protocols,” said Mendels. “But If MCP gains wide adoption as the first standard of its kind, we could see techniques and best practices begin to standardize around it.”
Can Anthropic MCP Set the Standard for Context-Aware AI Integration?
Despite its potential, Anthropic MCP faces significant challenges. Security is a primary concern, as enabling AI systems to access sensitive enterprise data raises the risk of leaks if the system goes rogue. Moreover, convincing developers already entrenched in established ecosystems to adopt MCP could prove difficult.
Another issue is the sheer size of the data, according to JD Raimondi, head of data science at IT development firm Making Sense. He told me, “Anthropic is the leader in experiments leading to large contexts, but the accuracy of the models suffer greatly. It's likely that they'll get better over time, and performance-wise, there are lots of tricks to keep the speed acceptable.”
While Anthropic asserts that MCP improves AI's ability to retrieve and contextualize data, the lack of concrete benchmarks to support these claims may hinder adoption. “Whether you’re an AI tool developer, an enterprise looking to leverage existing data, or an early adopter exploring the frontier, we invite you to build the future of context-aware AI together,” said Anthropic.
As developers test MCP’s capabilities, the industry will be watching to see if this open standard can gain the traction needed to become a benchmark for context-aware AI integration. Mendels suggests that standardization could be a smart move for Anthropic, potentially boosting interoperability and allowing teams to experiment with different combinations of tools to determine the best fit for their needs. “Right now, it feels too early to say that many processes in the AI ecosystem are standardizing,” Mendels noted. “With innovation happening so rapidly, today’s best practices might be outdated by next week. Only time will tell if a protocol like MCP can succeed in standardizing context data retrieval.”
The post Breaking Data Barriers: Can Anthropic’s Model Context Protocol Enhance AI Performance? appeared first on Unite.AI.
What's Your Reaction?
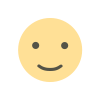
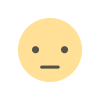
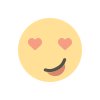
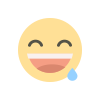
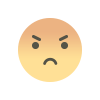
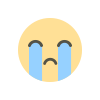
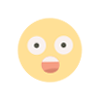