The Case for Healthcare-Specific Large Language Models
Large language models (LLMs) like ChatGPT and other general-purpose artificial intelligence (AI) systems have demonstrated remarkable versatility, excelling in tasks such as summarization, content generation, and conversational interfaces. However, when it comes to medicine, the limitations of general-purpose AI become glaringly apparent. Healthcare is uniquely complex, requiring a specialized approach to AI development. In fact, ... Read More
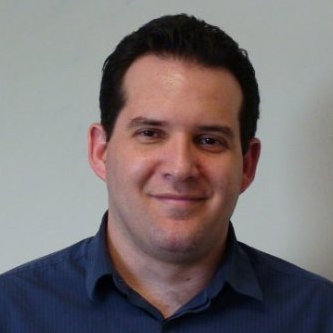
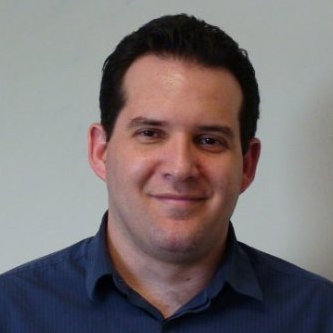
Large language models (LLMs) like ChatGPT and other general-purpose artificial intelligence (AI) systems have demonstrated remarkable versatility, excelling in tasks such as summarization, content generation, and conversational interfaces. However, when it comes to medicine, the limitations of general-purpose AI become glaringly apparent.
Healthcare is uniquely complex, requiring a specialized approach to AI development. In fact, there is overwhelming evidence from academic research and industry benchmarks that domain-specific and task-specific large language models outperform general-purpose LLMs across multiple dimensions. The moral of the story? Not all AI is created equally, and healthcare is a prime example why. Here are some important considerations for practitioners.
Medicine vs. Other Regulated Industries
Finance and law are other highly regulated fields where AI is playing an increasingly important role. However, while these industries deal with intricate processes, extensive regulations, and large data sets, healthcare presents an even greater challenge due to the sheer complexity of humans and our healthcare systems. With the nuanced nature of medical language and the ethical stakes involved, accuracy is key.
As a result, healthcare is one area where domain-specific LLMs outperform general-purpose LLMs. This is in contrast to other regulated domains like finance or law, perhaps because medicine is larger, more complex, and continuously evolving in a way that other domains are not. Whatever the underlying cause, the evidence is clear: Healthcare-specific LLMs outperform general-purpose LLMs on both public benchmarks like OpenMed and in real-world implementations. This has been the case consistently since transformers were introduced.
Challenges of Healthcare AI
One of the primary reasons general-purpose AI struggles in healthcare is the distinct nature of medical language. Medical terminology is not only highly specialized but also context-dependent. The same term can have different meanings based on the medical specialty, patient history, or even regional practices.
For instance, the abbreviation “RA” could mean rheumatoid arthritis to a rheumatologist, but to a cardiologist, it might mean right atrium. Similarly, drug interactions and dosages are highly specific to patient physiology, comorbidities, and genetic factors. General-purpose LLMs trained on broad datasets may not have the necessary depth of understanding to accurately interpret and apply medical knowledge without significant fine-tuning.
Medicine also relies heavily on implicit knowledge and unstructured data. Clinical notes, for example, contain shorthand, abbreviations, and informal language that may not be well-represented in generic AI models. A healthcare-specific LLM must be trained on vast amounts of domain-specific data, including electronic health records (EHRs), peer-reviewed medical literature, and real-world clinical dialogues, to ensure accurate comprehension and decision support.
The Need for Healthcare-Specific LLMs
Given these challenges, healthcare practitioners require AI systems built specifically for their domain. Healthcare-specific LLMs are trained on medical texts, patient records, imaging, and physician interactions to develop a deeper understanding of the field. These models are designed to recognize clinical nuances, understand contextual meanings, and provide relevant insights that align with current medical best practices.
Such models are already making a difference in areas like radiology, pathology, and drug discovery. AI-powered diagnostic tools assist radiologists in detecting abnormalities in medical imaging with higher accuracy, while AI-driven research platforms help identify potential drug candidates faster than traditional methods. Let’s not forget the operations side—healthcare specific LLMs have the power to predict appropriate staffing levels and help streamline back-end tasks, like billing insurance.
However, ensuring these models meet rigorous medical standards requires careful curation of training data, adherence to constantly-changing regulatory frameworks, and continuous validation by domain experts, which brings us to the next challenge:
Ethical and Regulatory Considerations
Another key reason healthcare AI must be distinct from general-purpose AI is the ethical and regulatory landscape. The healthcare industry operates under strict guidelines, such as HIPAA in the US and GDPR in Europe, which govern the use of patient data. Any AI system handling sensitive health information must comply with these regulations, ensuring robust security, privacy, and explainability.
Furthermore, transparency in AI decision-making is critical in medicine. A financial AI model that recommends an investment strategy can afford to be a “black box” to some extent, as long as it delivers strong results. In contrast, a healthcare AI model that assists in diagnosing cancer or recommending treatment options must be fully interpretable so that doctors can understand and validate its reasoning before making clinical decisions.
Bias is another major concern. General-purpose LLMs trained on internet data may reflect biases present in those datasets, leading to disparities in AI-driven healthcare recommendations. Healthcare-specific models must be trained on diverse, representative medical data to ensure they serve all patient populations fairly and equitably.
Domain Specific Models vs. Open AI
A recent blind evaluation by practicing medical doctors compared GPT-4o, trained by OpenAI, and a “small” medical LLM, MedS. The results demonstrated that due to its domain-specific data and task specialization, the medical LLM outperformed GPT-4o in the measured tasks—even being two orders of magnitude smaller. Another benefit of the smaller model is that it can be deployed on-premise, simplifying privacy and compliance healthcare companies must adhere to.
Clinicians preferred the outputs of the Medical LLM nearly 2x more often than GPT-4o on tasks including clinical text summarization, clinical information extraction, and biomedical question answering. Clinicians were asked to decide which option they preferred (between two blinded options) on three dimensions: factuality, clinical relevance, and conciseness. The medical LLM was heavily preferred across all three dimensions.
Evaluations included questions such as summarizing a patient’s medical history, summarizing the primary diagnosis, follow-up after surgery, or asking if a certain treatment seemed effective. These results underscore the value of domain-specific fine-tuning for improving model performance in specialized fields.
A Look Ahead
The future of AI in healthcare depends on the development of domain-specific models that prioritize accuracy, transparency, and patient safety. Rather than relying on one-size-fits-all AI solutions, it’s imperative for healthcare users to invest in specialized LLMs designed to meet the unique demands of medical practice.
While general-purpose AI is transforming many industries, healthcare stands alone in its complexity, language, and ethical and regulatory considerations. To fully realize the potential of AI in medicine, we must embrace the need for healthcare-specific AI because precision is not just a luxury; it is a necessity.
About David Talby
David Talby, Ph.D., MBA, is the CEO of John Snow Labs. He has spent his career making AI, big data, and data science solve real-world problems in healthcare, life science, and related fields. John Snow Labs is an award-winning AI for healthcare company providing state-of-the-art software, models, and data that power the world’s leading pharmaceuticals, academic medical centers, and health technology companies. Creator and host of The Healthcare NLP Summit, the company is committed to further educating and advancing the global healthcare and AI communities.