Step Towards Best Practices for Open Datasets for LLM Training
Large language models rely heavily on open datasets to train, which poses significant legal, technical, and ethical challenges in managing such datasets. There are uncertainties around the legal implications of using data based on varying copyright laws and changing regulations regarding safe usage. The lack of global standards or centralized databases to validate and license […] The post Step Towards Best Practices for Open Datasets for LLM Training appeared first on MarkTechPost.



Large language models rely heavily on open datasets to train, which poses significant legal, technical, and ethical challenges in managing such datasets. There are uncertainties around the legal implications of using data based on varying copyright laws and changing regulations regarding safe usage. The lack of global standards or centralized databases to validate and license datasets and incomplete or inconsistent metadata makes it impossible to assess the legal status of works. Technical barriers also relate to access to digitized public domain material. Most open datasets are not governed and have not implemented any kind of legal safety net for their contributors, exposing them to dangers and making them impossible to scale up. While intended to create more transparency and collaborative work, they do little or nothing to engage broader social challenges such as diversity and accountability and often exclude underrepresented languages and viewpoints.
Current methods of building open datasets for LLMs often lack clear legal frameworks and face significant technical, operational, and ethical challenges. Traditional methods depend on incomplete metadata, complicating verifying copyright status and compliance across different regions with different laws. Digitization of public domain materials and making them accessible is challenging because big projects like Google Books restrict usage, which prevents the construction of open datasets. Volunteer-driven projects lack structured governance, which exposes the contributors to legal risks. Such gaps prevent equal access, prevent diversity in data representation, and concentrate power in a few dominant organizations. This creates an ecosystem where open datasets struggle to compete with proprietary models, reducing accountability and slowing progress toward transparent and inclusive AI development.
To mitigate issues in metadata encoding, data sourcing, and processing for machine learning datasets, researchers proposed a framework focused on building a reliable corpus using openly licensed and public domain data for training large language models (LLMs). The framework emphasizes overcoming technical challenges like ensuring reliable metadata and digitizing physical records. It promotes cross-domain cooperation to responsibly curate, govern, and release these datasets while promoting competition in the LLM ecosystem. It also emphasizes metadata standards, reproducibility for accountability, and ensuring data source diversity as an alternative to more traditional methods lacking structured governance and transparency.
Researchers included all the practical steps of sourcing, processing, and governing datasets. Tools for detecting openly licensed content were used to ensure high-quality data. The framework integrated standards for metadata consistency, emphasized digitization, and encouraged collaboration with communities to create datasets. It also supported transparency and reproducibility in preprocessing and addressed potential biases and harmful content in a robust and inclusive system for training LLMs while reducing legal risks. The framework also highlights engaging with underrepresented communities to build diverse datasets and create clearer, machine-readable terms of Use. Additionally, making the open data ecosystem sustainable should come through proposed funding models on public funding from both tech companies and cultural institutions to ensure sustainable participation.
Finally, the researchers provided a clear scenario with a broadly outlined plan on how to approach the issues discussed within the context of training LLMs on non-licensed data, with a focus on the openness of the datasets and the efforts made by different spheres. Initiatives such as emphasizing metadata standardization, enhancing the digitization process, and responsible governance were intended to make the artificial intelligence ecosystem more open. The works build the foundation for future works where further probing into newer innovations in dataset management, AI governance, and advancements of the technologies that enhance the accessibility of data while addressing the problem of ethical and legal challenges.
Check out the Paper. All credit for this research goes to the researchers of this project. Also, don’t forget to follow us on Twitter and join our Telegram Channel and LinkedIn Group. Don’t Forget to join our 65k+ ML SubReddit.
What's Your Reaction?
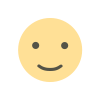
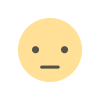
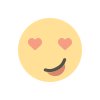
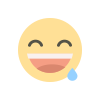
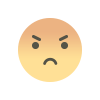
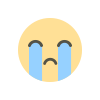
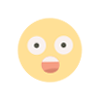