In 2025, GenAI Copilots Will Emerge as the Killer App That Transforms Business and Data Management
Every technological revolution has a defining moment when a specific use case propels the technology into widespread adoption. That time has come for generative AI (GenAI) with the rapid spread of copilots. GenAI as a technology has taken significant strides in the past few years. Yet despite all the headlines and hype, its adoption by […] The post In 2025, GenAI Copilots Will Emerge as the Killer App That Transforms Business and Data Management appeared first on Unite.AI.
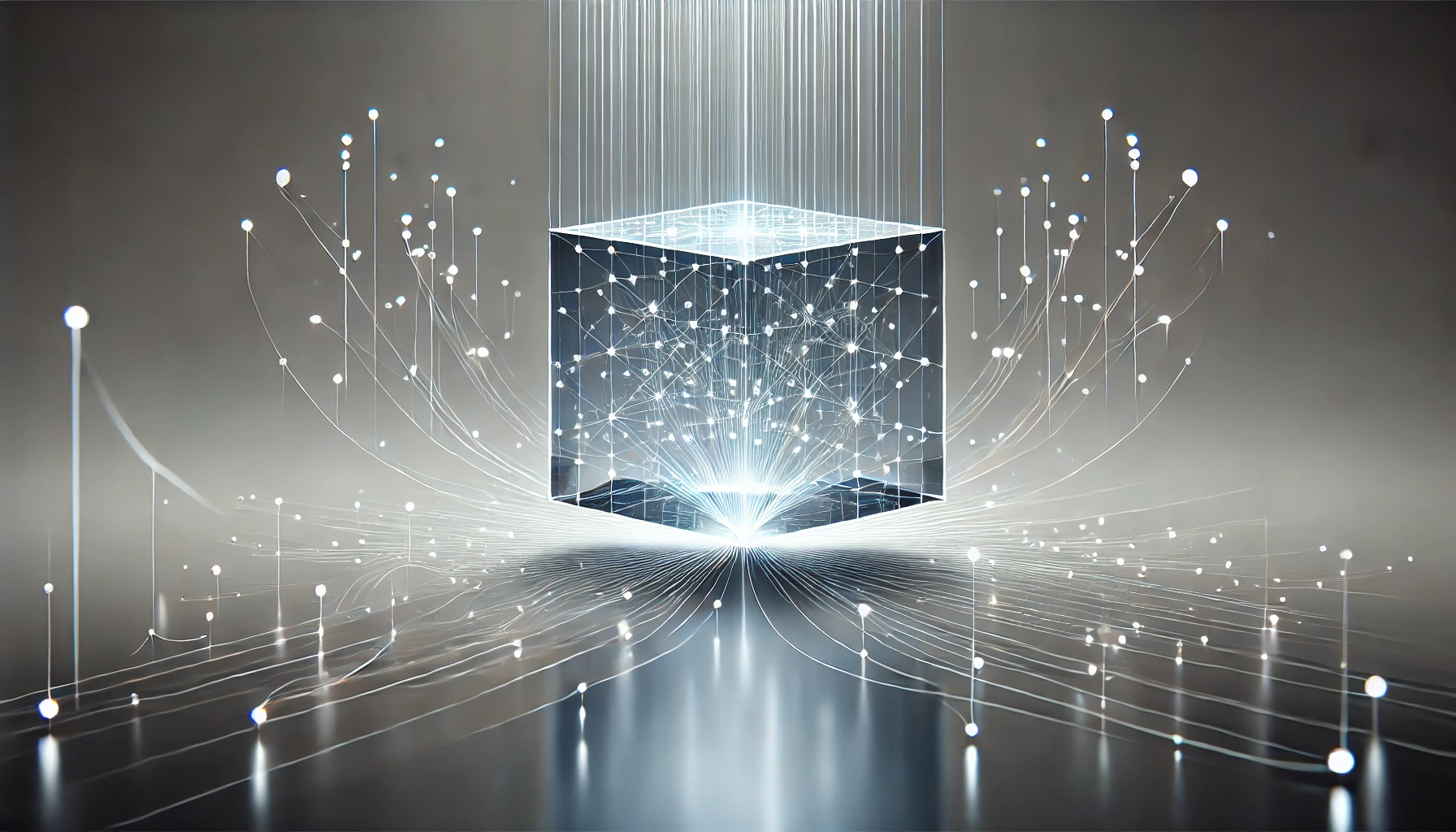

Every technological revolution has a defining moment when a specific use case propels the technology into widespread adoption. That time has come for generative AI (GenAI) with the rapid spread of copilots.
GenAI as a technology has taken significant strides in the past few years. Yet despite all the headlines and hype, its adoption by companies is still in the early stages. The 2024 Gartner CIO and Tech Executive Survey puts adoption at only 9% of those surveyed, with 34% saying they plan to do so in the next year. A recent survey by the Enterprise Strategy Group puts GenAI adoption at 30%. But the surveys all come to the same conclusion about 2025.
Prediction 1. A Majority of Enterprises Will Use GenAI in Production by the End of 2025
GenAI adoption is seen as critical to improving productivity and profitability and has become a top priority for most businesses. But it means that companies must overcome the challenges experienced so far in GenAII projects, including:
- Poor data quality: GenAI ends up only being as good as the data it uses, and many companies still don’t trust their data. Data quality along with incomplete or biased data have all been issues that lead to poor results.
- GenAI costs: training GenAI models like ChatGPT has mostly only been done by the very best of the best GenAI teams and costs millions in computing power. So instead people have been using a technique called retrieval augmented generation (RAG). But even with RAG, it quickly gets expensive to access and prepare data and assemble the experts you need to succeed.
- Limited skill sets: Many of the early GenAI deployments required a lot of coding by a small group of experts in GenAI. While this group is growing, there is still a real shortage.
- Hallucinations: GenAI isn’t perfect. It can hallucinate, and give wrong answers when it thinks it’s right. You need a strategy for preventing wrong answers from impacting your business.
- Data security: GenAI has exposed data to the wrong people because it was used for training, fine-tuning, or RAG. You need to implement security measures to protect against these leaks.
Luckily the software industry has been tackling these challenges for the past few years. 2025 looks like the year when several of these challenges start to get solved, and GenAI becomes mainstream.
Prediction 2. Modular RAG Copilots Will Become The Most Common Use of GenAI
The most common use of GenAI is to create assistants, or copilots, that help people find information faster. Copilots are usually built using RAG pipelines. RAG is the Way. It’s the most common way to use GenAI. Because Large Language Models (LLM) are general-purpose models that don’t have all or even the most recent data, you need to augment queries, otherwise known as prompts, to get a more accurate answer.
Copilots help knowledge workers be more productive, address previously unanswerable questions, and provide expert guidance while sometimes also executing routine tasks. Perhaps the most successful copilot use case to date is how they help software developers code or modernize legacy code.
But copilots are expected to have a bigger impact when used outside of IT. Examples include:
- In customer service, copilots can receive a support query and either escalate to a human for intervention or provide a resolution for simple queries like password reset or account access, resulting in higher CSAT scores.
- In manufacturing, co-pilots can help technicians diagnose and recommend specific actions or repairs for complex machinery, reducing downtime.
- In healthcare, clinicians can use copilots to access patient history and relevant research and help guide diagnosis and clinical care, which improves efficiency and clinical outcomes.
RAG pipelines have mostly all worked the same way. The first step is to load a knowledge base into a vector database. Whenever a person asks a question, a GenAI RAG pipeline is invoked. It re-engineers the question into a prompt, queries the vector database by encoding the prompt to find the most relevant information, invokes an LLM with the prompt using the retrieved information as context, evaluates and formats the results, and displays them to the user.
But it turns out you can’t support all copilots equally well with a single RAG pipeline. So RAG has evolved into a more modular architecture called modular RAG where you can use different modules for each of the many steps involved:
- Indexing including data chunking and organization
- Pre-retrieval including query (prompt) engineering and optimization
- Retrieval with retriever fine-tuning and other techniques
- Post-retrieval reranking and selection
- Generation with generator fine-tuning, using and comparing multiple LLMs, and verification
- Orchestration that manages this process, and makes it iterative to help get the best results
You will need to implement a modular RAG architecture to support multiple copilots.
Prediction 3. No-Code/Low-Code GenAI Tools Will Become The Way
By now, you may realize GenAI RAG is very complex and rapidly changing. It’s not just that new best practices are constantly emerging. All the technology involved in GenAI pipelines is changing so fast that you will end up needing to swap out some of them or support several. Also, GenAI isn’t just about modular RAG. Retrieval Augmented Fine Tuning (RAFT) and full model training are becoming cost-effective as well. Your architecture will need to support all this change and hide the complexity from your engineers.
Thankfully the best GenAI no-code/low-code tools provide this architecture. They are constantly adding support for leading data sources, vector databases, and LLMS, and making it possible to build modular RAG or feed data into LLMs for fine-tuning or training. Companies are successfully using these tools to deploy copilots using their internal resources.
Nexla doesn’t just use GenAI to make integration simpler. It includes a modular RAG pipeline architecture with advanced data chunking, query engineering, reranking and selection, multi-LLM support with results ranking and selection, orchestration, and more – all configured without coding.
Prediction 4. The Line between Copilots and Agents Will Blur
GenAI copilots like chatbots are agents that support people. In the end people make the decision on what to do with the generated results. But GenAI agents can fully automate responses without involving people. These are often referred to as agents or agentic AI.
Some people view these as two separate approaches. But the reality is more complicated. Copilots are already starting to automate some basic tasks, optionally allowing users to confirm actions and automating the steps needed to complete them.
Expect copilots to evolve over time into a combination of copilots and agents. Just like applications help re-engineer and streamline business processes, assistants could and should start to be used to automate intermediate steps of the tasks they support. GenAI-based agents should also include people to handle exceptions or approve a plan generated using an LLM.
Prediction 5. GenAI Will Drive The Adoption of Data Fabrics, Data Products, and Open Data Standards
GenAI is expected to be the biggest driver of change in IT over the next few years because IT will need to adapt to enable companies to realize the full benefit of GenAI.
As part of the Gartner Hype Cycles for Data Management, 2024, Gartner has identified 3, and only 3 technologies as transformational for data management and for the organizations that depend on data: Data Fabrics, Data Products, and Open Table Formats. All 3 help make data much more accessible for use with GenAI because they make it easier for data to be used by these new sets of GenAI tools.
Nexla implemented a data product architecture built on a data fabric for this reason. The data fabric provides a unified layer to manage all data the same way regardless of differences in formats, speeds, or access protocols. Data products are then created to support specific data needs, such as for RAG.
For example, one large financial services firm is implementing GenAI to enhance risk management. They’re using Nexla to create a unified data fabric. Nexla automatically detects schema and then generates connectors and data products. The company then defines data products for specific risk metrics that aggregate, cleanse, and transform data into the right format as inputs implementing RAG agents for dynamic regulatory reporting. Nexla provides the data governance controls including data lineage and access controls to ensure regulatory compliance.Our integration platform for analytics, operations, B2B and GenAI is implemented on a data fabric architecture where GenAI is used to create reusable connectors, data products, and workflows. Support for open data standards like Apache Iceberg makes it easier to access more and more data.
How to Copilot Your Way Towards Agentic AI
So how should you get ready to make GenAI mainstream in your company based on these predictions?
First, if you haven’t yet, get started on your first GenAI RAG assistant for your customers or employees. Identify an important, and relatively straightforward use case where you already have the right knowledgebase to succeed.
Second, make sure to have a small team of GenAI experts who can help put the right modular RAG architecture, with the right integration tools in place to support your first projects. Don’t be afraid to evaluate new vendors with no-code/low-code tools.
Third, start to identify those data management best practices that you will need to succeed. This not only involves a data fabric and concepts like data products. You also need to govern your data for AI.
The time is now. 2025 is the year the majority will succeed. Don’t get left behind.
The post In 2025, GenAI Copilots Will Emerge as the Killer App That Transforms Business and Data Management appeared first on Unite.AI.