Cycle Time is the New Currency of Drug Development
For years, the life sciences industry has been trying to address the same R&D challenges: rising costs, patient recruitment and retention, and difficulty maximizing ROI. Drug development is expensive, and failure rates remain high. Studies estimate the total cost to develop a new drug is between $300 million and nearly $4.5 billion. Meanwhile, clinical trial ... Read More
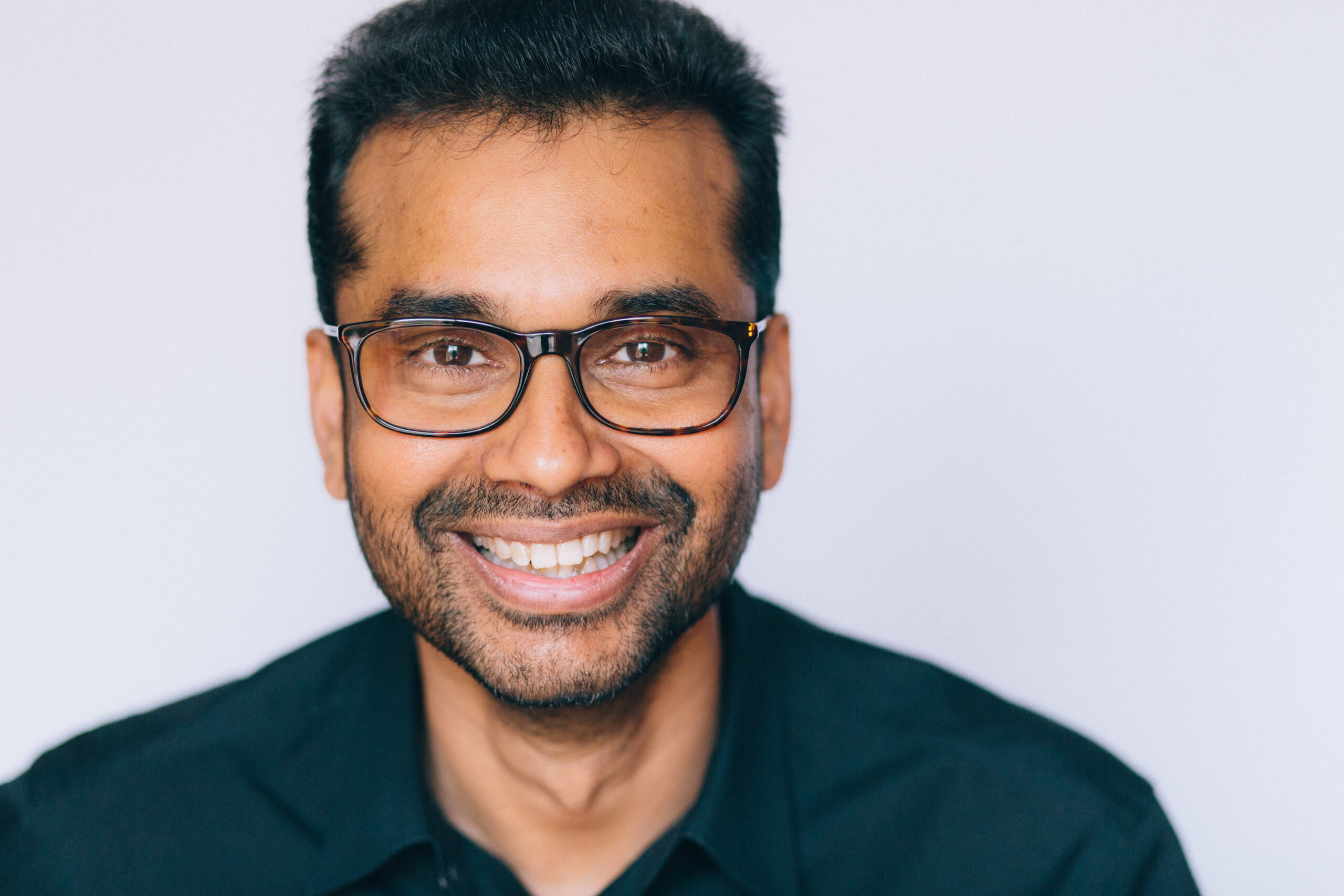
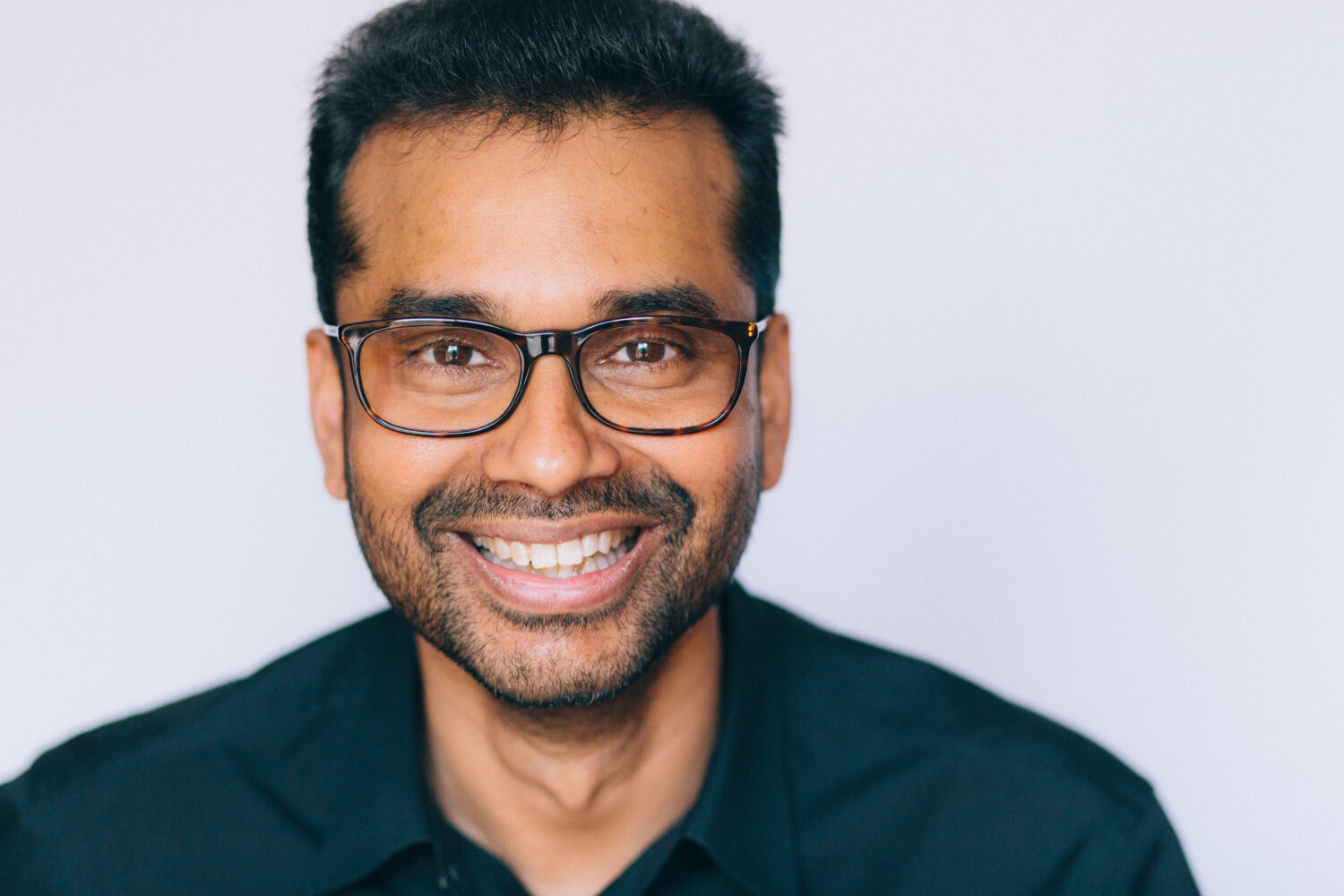
For years, the life sciences industry has been trying to address the same R&D challenges: rising costs, patient recruitment and retention, and difficulty maximizing ROI. Drug development is expensive, and failure rates remain high. Studies estimate the total cost to develop a new drug is between $300 million and nearly $4.5 billion. Meanwhile, clinical trial cycle times continue to lengthen – significantly impacting overall drug development timelines. Clinical trials are increasingly complex, with more complicated protocols and more data collected than ever before. The data points collected in Phase III pivotal trials increased by 283.2% in the decade between 2010 and 2020, according to the Tufts Center for the Study of Drug Development. Tufts data also shows expanding complexity of protocol designs correlates to worsening clinical trial performance, including reduced data quality, escalating costs, and extended cycle times.
I have spent more than 25 years in clinical research technology, and during this time, my industry peers and I have been discouraged by these unchanging statistics, despite years of effort to address them. The urgency of the situation is palpable leading to several questions. How can clinical trials support groundbreaking scientific discoveries and quickly bring novel science to patients? How can clinical research be faster, more efficient, and more flexible? As it stands now, we are caught in a vicious cycle, where not only are patients waiting for years for life-saving treatments, but the treatments that make it to market are priced to compensate for the bloat and waste in this lengthy and inefficient process. The industry-wide inefficiency problem needs to be addressed more aggressively. It is imperative to reduce costs and accelerate market access. Cycle time is the new currency of drug development, and we must act now.
The rapid advancement of AI stands in stark contrast to the stagnant cycle time of drug development. AI and ML breakthroughs have significantly impacted the life sciences, especially drug discovery, and AI has been a topic of discussion for many years. While machine learning has existed for decades, recent breakthroughs, particularly in generative AI (GenAI), are happening at a breathtaking pace and are opening up possibilities that could not have been envisioned a few months ago. For example, image classification took years to reach human-level performance, but GenAI models, using techniques like multitask language understanding, surpassed human capabilities in mere months. These models like OpenAI’s ChatGPT can now solve complex math problems, providing human-like reasoning and step-by-step explanations. In some instances, they even outperform PhD-level scientists.
AI is transforming a wide range of industries and functions, including coding, customer support, and marketing. Given the significant impact AI is having, companies across various sectors are beginning to rethink their operational strategies to adapt to an AI-driven landscape. The field of drug development should be no exception and should embrace an AI-first approach to drive future innovation and success.
The good news is that there is sustained interest in AI development and research, indicating that our industry will continue to invest in AI for increased value. There’s a widespread understanding of the potential for technology to automate and reduce cycle times. However, compared to other industries, AI adoption is slow in biopharma. This is due to skepticism that decades-old, pervasive industry challenges can be solved simply by applying new technology.
In the “pre-GenAI” era, the advancement of clinical technology did not bridge the gap between industry challenges and outcomes, leading to skepticism around ROI for large-scale transformative initiatives. For example, life sciences R&D has made significant strides specifically in leveraging modern data infrastructure and analytics for faster insights and better decision-making. There are still several manual processes in the overall drug development creating friction and leading to operational inefficiencies. Data silos continue to persist, with sponsors struggling to gain control of their data and extract value from their most valuable asset: data.
Now that we have technology that continues to change at an unimaginable pace, with ML models performing various tasks efficiently and the rise of AI agents overseeing entire workflows, it necessitates a new approach to adoption. Enterprises must embrace reinvention rather than incremental transformation to leverage these opportunities. We are amidst an AI super cycle, where capabilities are evolving at an unprecedented pace. Applied effectively, AI can solve significant industry challenges. In clinical data management, AI can be integrated from acquisition to submission and insight generation. The emergence of agentic AI further expands these possibilities, with specialized agents from different systems communicating and eliminating inefficiencies, making our envisioned future a reality.
Unlocking AI’s potential requires embedding it pervasively across the clinical data lifecycle. Reinvention is essential to bridge the chasm between challenges and outcomes. Broader improvements will not come from AI by automating discrete processes within the entire value chain or by implementing incremental changes. Life sciences R&D leaders must take a step back and look at the possibilities with a reinvention mindset.
To fully leverage AI, businesses need to reimagine it as middleware – a connective layer between data and applications. This strategic shift necessitates a fundamental redesign of workflows, integrating AI capabilities across the entire value chain to close the gap between AI’s potential and existing processes. Taking this approach could finally unlock the value of widespread cycle time reduction.
The life sciences industry has been dealing with the same challenges and has not successfully leveraged technology advancements to address them. These problems are becoming more complex, resulting in increased cycle times. Meanwhile, patients are waiting for new treatments while the industry grapples with recurrent themes. For this reason, cycle time should be the new currency. Now, there is a unique opportunity to take an AI-first approach and think of reinvention across the end-to-end clinical research lifecycle. This reinvention strategy will optimize cycle times and future-proof the industry against emerging challenges in modern drug development, and deliver innovative therapies to patients faster.
About Raj Indupuri
Raj Indupuri is the CEO and Co-Founder of eClinical Solutions, responsible for establishing its vision and future-looking technology strategy. A technologist with over 25 years of industry experience, he is deeply passionate about fostering innovation to revolutionize the Life Sciences industry with ground-breaking technologies that will modernize clinical trials and bring treatments to patients faster. Raj is a Mechanical Engineer with an MBA from Boston University who firmly believes data is the new fuel that will drive human progress.