Ai2 Launches Tülu3-405B Model, Scales Reinforcement Learning for Open Source AI
This week, the Allen Institute for AI (Ai2) launched Tülu3-405B, a massive 405-billion parameter open source AI model claimed to outperform DeepSeek-V3 and match GPT-4o in key benchmarks, particularly mathematical reasoning and safety. This release showcases Ai2’s novel training method, Reinforcement Learning with Verifiable Rewards (RLVR). Tulu3-405B builds on Ai2’s Tulu3 post-training recipe, first introduced in November 2024. The model fine-tunes Meta’s Llama-405B using a combination of carefully curated data, supervised fine-tuning, Direct Preference Optimization (DPO), and RLVR. RLVR is particularly noteworthy because it enhances skills where verifiable outcomes exist, such as math problem-solving and instruction-following. According to Ai2’s findings, RLVR scaled more effectively at 405B parameters compared to smaller models like Tulu3-70B and Tulu3-8B. Scaling up gave Tulu3-405B a big boost in math skills, adding weight to the idea that bigger models do better when fed specialized data instead of a little bit of everything, a la broad datasets. Ai2’s internal evaluations suggest that Tulu3-405B consistently outperforms DeepSeek-V3, particularly in safety benchmarks and mathematical reasoning. The model also competes with OpenAI’s GPT-4o. Tulu3-405B also surpasses previous open-weight post-trained models, including Llama 3.1 405B Instruct and Nous Hermes 3 405B. Training a 405-billion parameter model is not a small task. Tulu3-405B required 256 GPUs across 32 nodes, using vLLM, an optimized inference engine, with 16-way tensor parallelism. According to a blog post, Ai2’s engineers faced several challenges, including these intense compute requirements: “Training Tülu 3 405B demanded 32 nodes (256 GPUs) running in parallel. For inference, we deployed the model using vLLM with 16-way tensor parallelism, while utilizing the remaining 240 GPUs for training. While most of our codebase scaled well, we occasionally encountered NCCL timeout and synchronization issues that required meticulous monitoring and intervention,” the authors wrote. There were also hyperparameter tuning challenges: “Given the computational costs, hyperparameter tuning was limited. We followed the principle of “lower learning rates for larger models” consistent with prior practice with Llama models,” the Ai2 team said. With Tulu3-405B, Ai2 isn’t just releasing another open-source AI model. It’s making a statement about model training. By scaling up its RLVR method, Ai2 has not only built a model that can hold its own against top-tier AI like GPT-4o and DeepSeek-V3, but it argues an important idea: bigger models can get better when trained the right way. Training Tulu3-405B didn’t just throw more data at the problem but used specialized, high-quality data and thoughtful training techniques to improve it. But beyond the technical wins, Tulu3-405B highlights a bigger shift in AI: the fight to keep innovation open and accessible. While the biggest AI models are often locked behind corporate paywalls, Ai2 is betting on a future where powerful AI remains available for researchers, developers, and anyone curious enough to experiment. To that end, Ai2 has made Tulu3-405B freely available for research and experimentation, hosting it on Google Cloud (and soon, Vertex) and offering a demo through the Ai2 Playground.

This week, the Allen Institute for AI (Ai2) launched Tülu3-405B, a massive 405-billion parameter open source AI model claimed to outperform DeepSeek-V3 and match GPT-4o in key benchmarks, particularly mathematical reasoning and safety.
This release showcases Ai2’s novel training method, Reinforcement Learning with Verifiable Rewards (RLVR). Tulu3-405B builds on Ai2’s Tulu3 post-training recipe, first introduced in November 2024. The model fine-tunes Meta’s Llama-405B using a combination of carefully curated data, supervised fine-tuning, Direct Preference Optimization (DPO), and RLVR.
RLVR is particularly noteworthy because it enhances skills where verifiable outcomes exist, such as math problem-solving and instruction-following. According to Ai2’s findings, RLVR scaled more effectively at 405B parameters compared to smaller models like Tulu3-70B and Tulu3-8B. Scaling up gave Tulu3-405B a big boost in math skills, adding weight to the idea that bigger models do better when fed specialized data instead of a little bit of everything, a la broad datasets.
Ai2’s internal evaluations suggest that Tulu3-405B consistently outperforms DeepSeek-V3, particularly in safety benchmarks and mathematical reasoning. The model also competes with OpenAI’s GPT-4o. Tulu3-405B also surpasses previous open-weight post-trained models, including Llama 3.1 405B Instruct and Nous Hermes 3 405B.
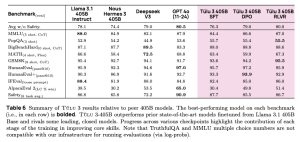
A table mapping Tülu 3 405B performance compared to other current models across several evaluation benchmarks. (Source: Ai2)
Training a 405-billion parameter model is not a small task. Tulu3-405B required 256 GPUs across 32 nodes, using vLLM, an optimized inference engine, with 16-way tensor parallelism. According to a blog post, Ai2’s engineers faced several challenges, including these intense compute requirements: “Training Tülu 3 405B demanded 32 nodes (256 GPUs) running in parallel. For inference, we deployed the model using vLLM with 16-way tensor parallelism, while utilizing the remaining 240 GPUs for training. While most of our codebase scaled well, we occasionally encountered NCCL timeout and synchronization issues that required meticulous monitoring and intervention,” the authors wrote.
There were also hyperparameter tuning challenges: “Given the computational costs, hyperparameter tuning was limited. We followed the principle of “lower learning rates for larger models” consistent with prior practice with Llama models,” the Ai2 team said.
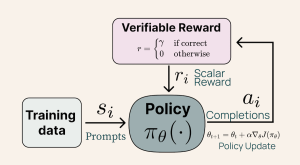
A diagram outlining the Reinforcement Learning with Verifiable Rewards (RLVR) process. (Source: Ai2)
With Tulu3-405B, Ai2 isn’t just releasing another open-source AI model. It’s making a statement about model training. By scaling up its RLVR method, Ai2 has not only built a model that can hold its own against top-tier AI like GPT-4o and DeepSeek-V3, but it argues an important idea: bigger models can get better when trained the right way. Training Tulu3-405B didn’t just throw more data at the problem but used specialized, high-quality data and thoughtful training techniques to improve it.
But beyond the technical wins, Tulu3-405B highlights a bigger shift in AI: the fight to keep innovation open and accessible. While the biggest AI models are often locked behind corporate paywalls, Ai2 is betting on a future where powerful AI remains available for researchers, developers, and anyone curious enough to experiment.
To that end, Ai2 has made Tulu3-405B freely available for research and experimentation, hosting it on Google Cloud (and soon, Vertex) and offering a demo through the Ai2 Playground.